- Bitergia
- Partnerships
- NumFOCUS Foundation
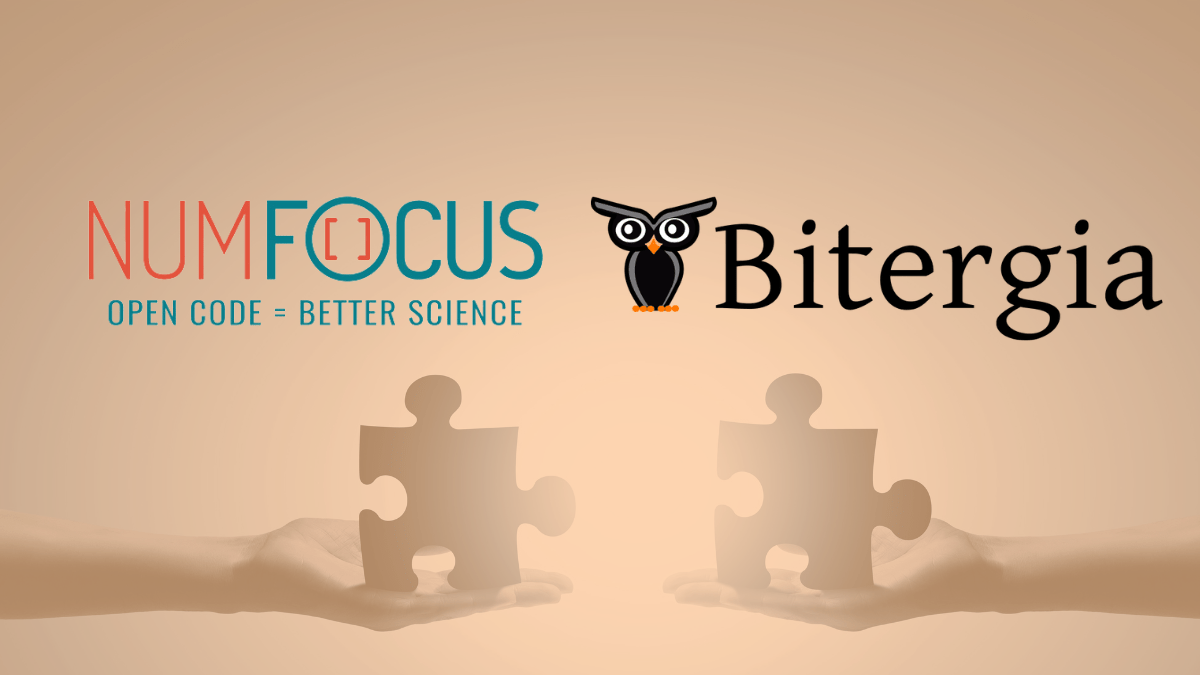
NumFOCUS have over 100 sponsored and affiliated projects. The goal for the next 2 years is to provide community metrics for all of these projects. This is a lot. As a first step, NumFOCUS and Bitergia identified 9 projects as early adopters. These early adopting projects are set up on a shared dashboard and can start to see metrics about their communities.
We are now discussing between these projects, NumFOCUS, and Bitergia about the specific setup of the dashboard. The goal is to identify which metrics and insights make sense for these NumFOCUS projects. We may build out custom dashboards and visualizations that can then be used by other NumFOCUS projects.
– Thierry Carrez, GM of the OpenInfra Foundation.
We look forward to exploring, what insights we can gain together about these projects. We’ll make sure to share these insights. As the new Official Metrics Partner, we plan to expand the community metrics dashboard to more NumFOCUS projects.
The 9 projects that are part of the pilot project include:
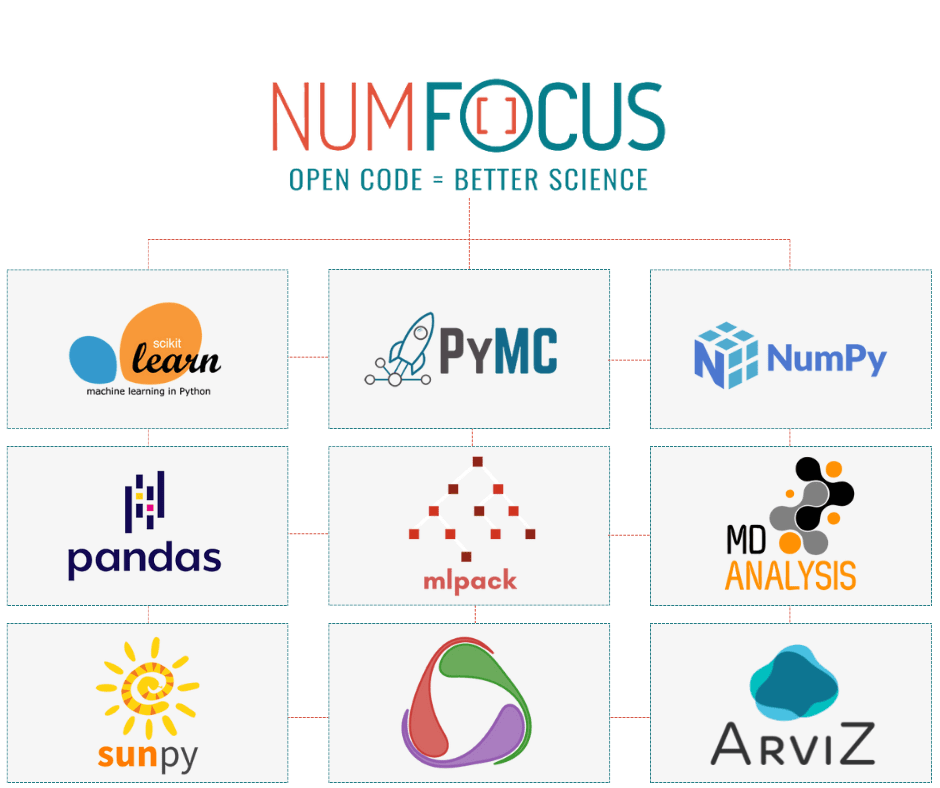
ArviZ is an open source project aiming to provide tools for the Exploratory Analysis of Bayesian Models that do not depend on the inference library used.
Go to: ArviZ website
MDAnalysis is a Python library for the analysis of computer simulations of many-body systems at the molecular scale, spanning use cases from interactions of drugs with proteins to novel materials. It is widely used in the scientific community and is written by scientists for scientists.
Go to: MDAnalysis website
mlpack is a fast, flexible machine learning library suitable for both data science prototyping and deployment.
Go to: mlpack website
pandas is a data wrangling platform for Python widely adopted in the scientific computing community. pandas provide easy-to-use data ingestion, transformation, and export functions.
Go to: pandas website
PyMC is a Python-based statistical modeling tool for Bayesian statistical modeling and Probabilistic Machine Learning which focuses on advanced Markov chain Monte Carlo and variational fitting algorithms.
Go to: PyMC website
NumPy is a universal data structure that fundamentally enables data analysis in numerical computing by permitting the exchange of data between algorithms. NumPy is a foundational project for the Python scientific computing stack.
Go to: NumPy website
scikit–learn is a Python library for machine learning, and is one of the most widely used tools for supervised and unsupervised machine learning. Scikit–learn provides an easy-to-use, consistent interface to a large collection of machine learning models, as well as tools for model evaluation and data preparation.
Go to: scikit-learn website
SciML is an open source software organization created to unify the packages for scientific machine learning. This includes the development of modular scientific simulation support software. They provide a modular, easily-extendable, and highly performant ecosystem for handling a wide variety of scientific simulations.
Go to: SciML website
SunPy is a Python-based software library that provides tools for performing research using direct observations of the Sun and Heliosphere.
Go to: SunPy website
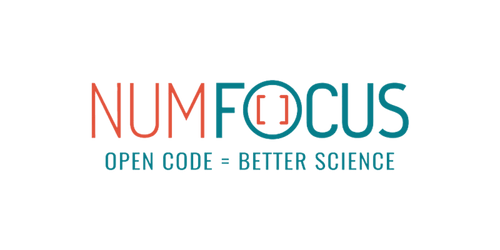
The mission of NumFOCUS is to promote open practices in research, data, and scientific computing by serving as a fiscal sponsor for open source projects and organizing community-driven educational programs.
In fact, to reach its vision and mission, NumFOCUS provides stewardship to its projects. By partnering with Bitergia to provide community metrics, NumFOCUS and Anaconda allow projects to understand their community health better. To build healthy and sustainable communities, the community metrics can help make decisions and also track the impact on the community.
Sustainable open source projects are important to reduce the risk of relying on open source software. This means fostering engaged communities that take responsibility for maintaining and advancing the software. Metrics provide key indicators about how a community is doing and where special attention may be needed. Key factors to evaluate include level of activity.
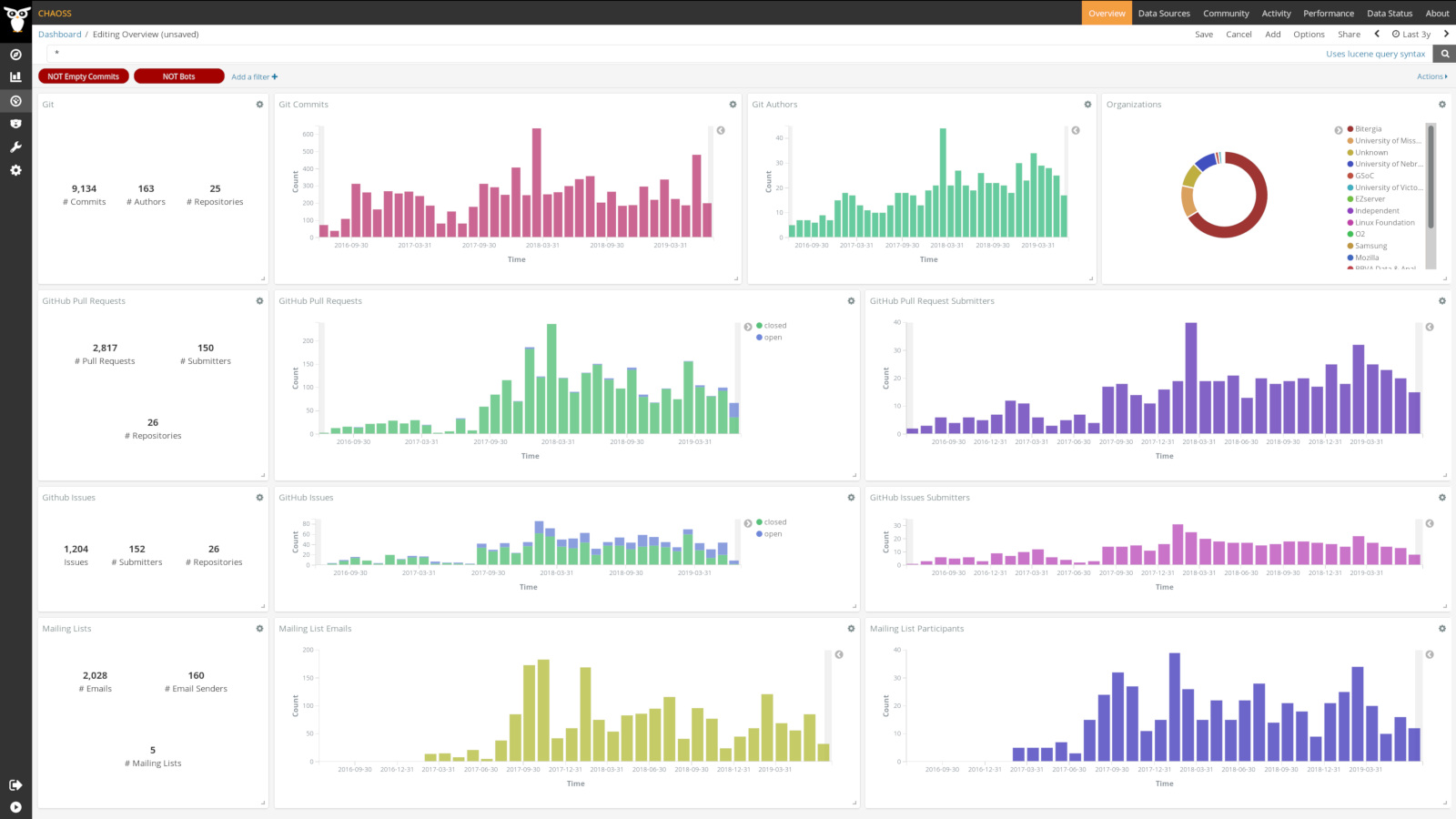
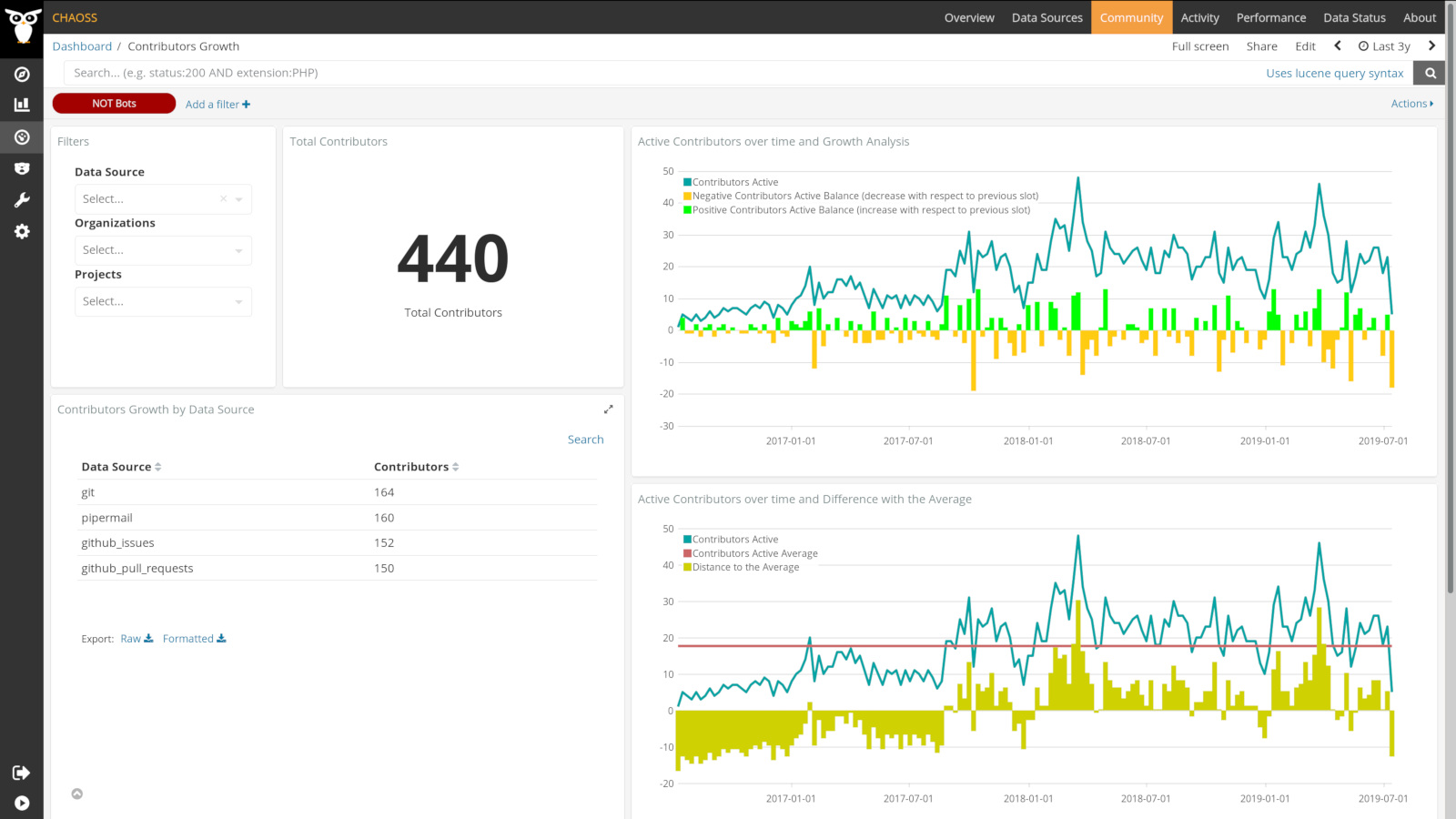
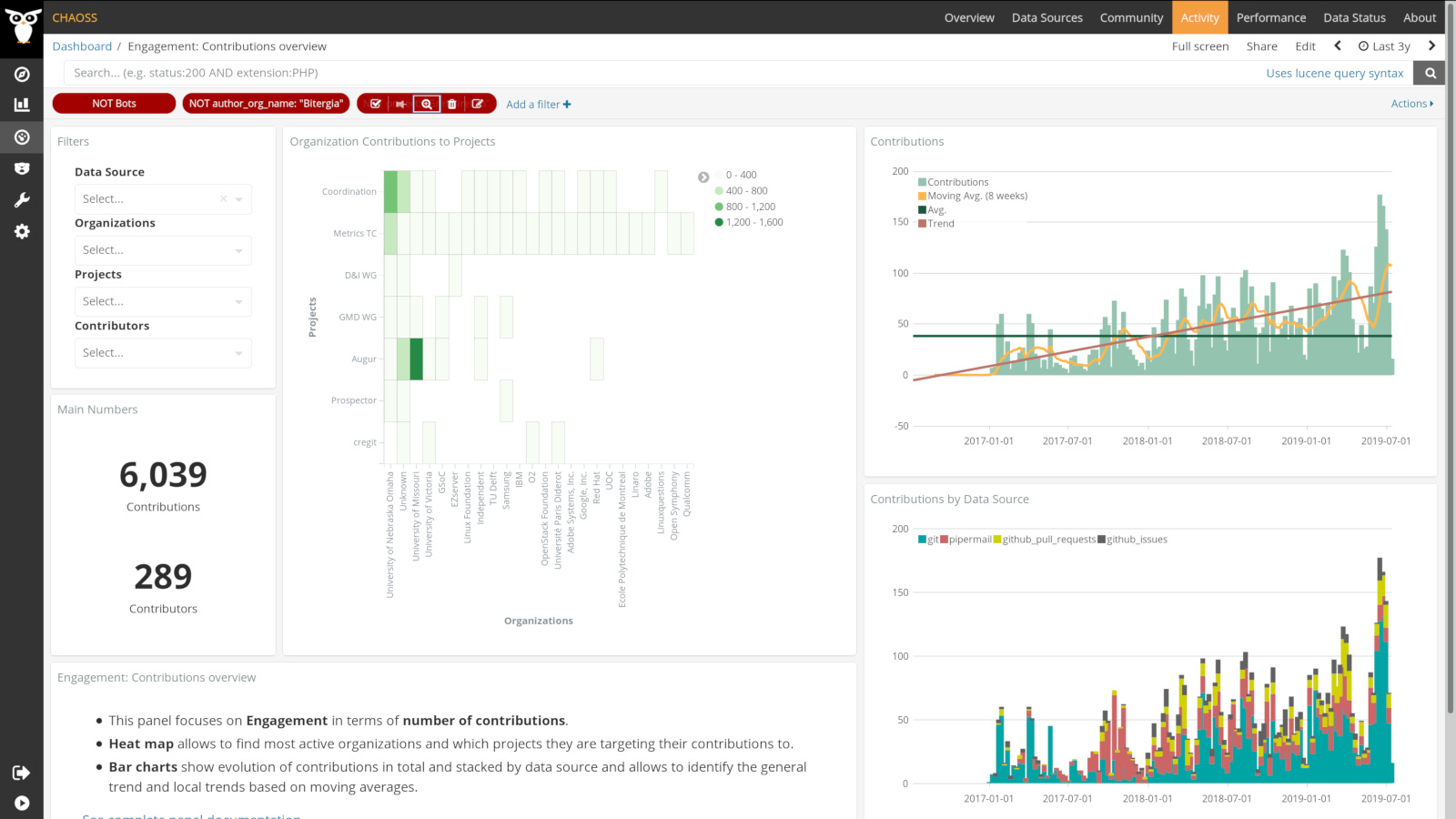
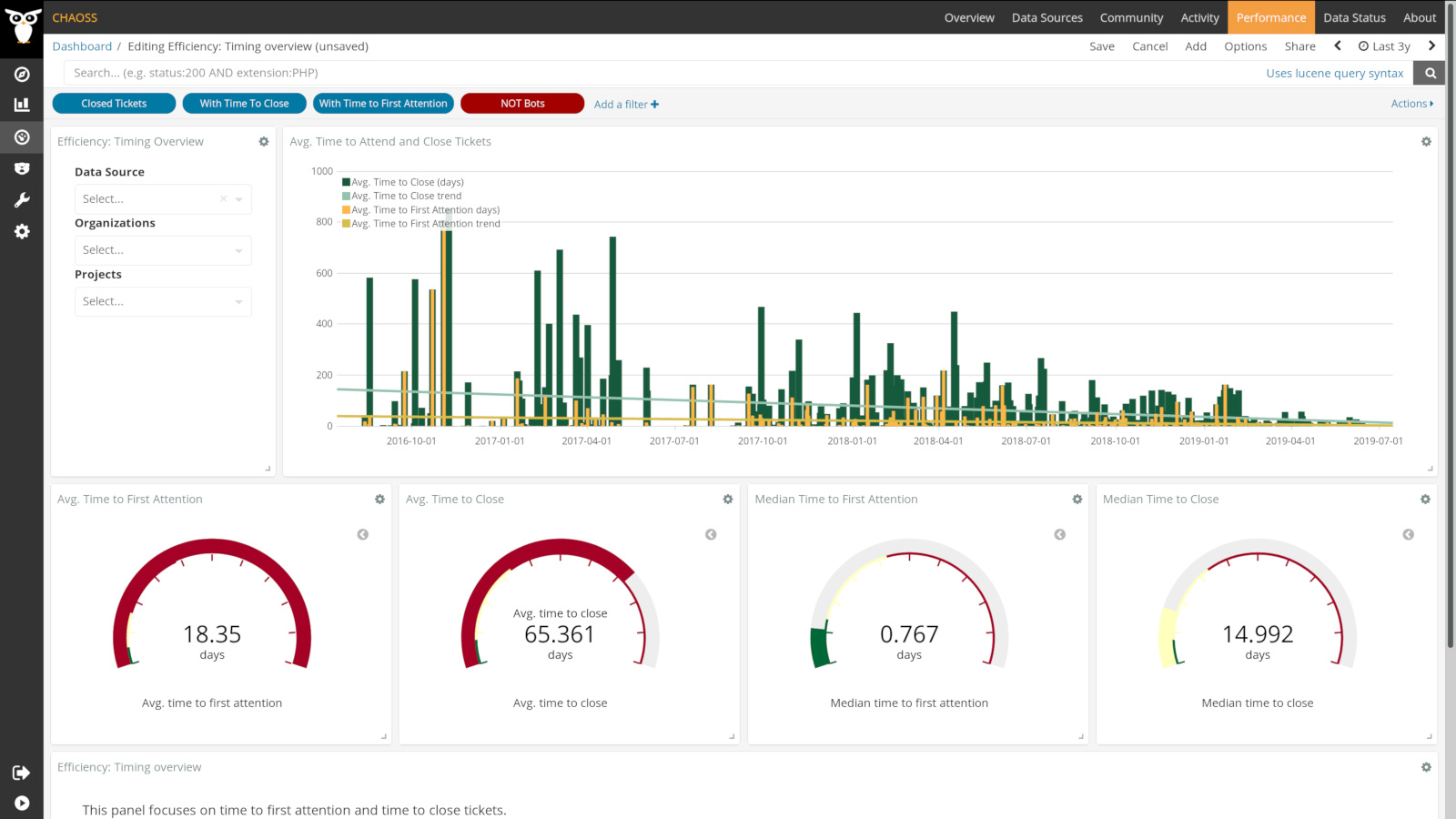